The Pros and Cons of AI in Product Management
AI is no longer just a buzzword—it’s become a central pillar in how businesses operate, innovate, and compete. Product management, being a field that thrives on data, insights, and decision-making, is naturally experiencing a major transformation thanks to AI. The fusion of artificial intelligence into product management processes has led to powerful efficiencies, smarter decision-making, and deeper customer understanding.
But here’s the kicker: it’s not all smooth sailing.
While AI is unlocking doors that were previously unimaginable, it also presents a slew of challenges that product managers can’t ignore. Think privacy issues, algorithmic bias, and the loss of that irreplaceable human instinct. This article dives deep into both sides of the coin—the good and the not-so-good—to give you a clear, honest look at what AI truly brings to the product management table.
So, should AI be your product manager’s best friend or a cautious ally? Let’s find out.
What is AI in Product Management?
Artificial intelligence in product management refers to the use of machine learning, natural language processing, and data analytics tools to enhance various aspects of the product development lifecycle. From concept to customer feedback, AI has found its place across the board.
Let’s break that down.
At its core, AI enables product teams to process vast amounts of data at lightning speed. Instead of relying solely on gut feelings or limited datasets, product managers can now leverage intelligent systems to inform their strategies. This means smarter decisions, faster development, and better outcomes.
Practical Applications of AI in Product Management
- Customer Feedback Analysis: Tools like MonkeyLearn and Thematic use NLP to sort through customer reviews and support tickets, giving product managers a clearer picture of user sentiment.
- Feature Prioritization: AI can analyze usage data, customer needs, and business goals to suggest what features to build next.
- Product Roadmapping: Platforms such as Craft.io or airfocus integrate AI to offer data-driven roadmaps tailored to strategic objectives.
- Predictive User Behavior: With AI, product teams can forecast how users will interact with features—before they even build them.
Common AI-Powered Tools for Product Managers
- Airtable with Automations – for streamlined workflows
- Amplitude & Mixpanel – for user behavior insights
- Notion AI – to speed up documentation and ideation
- Gainsight PX – for customer feedback and journey tracking
- Pendo – combining analytics and in-app messaging with AI-driven suggestions
- Peerbie – for AI-enhanced task and team management
In essence, AI tools are becoming essential allies, helping product managers make informed decisions, manage their time better, and uncover insights they’d likely miss otherwise.
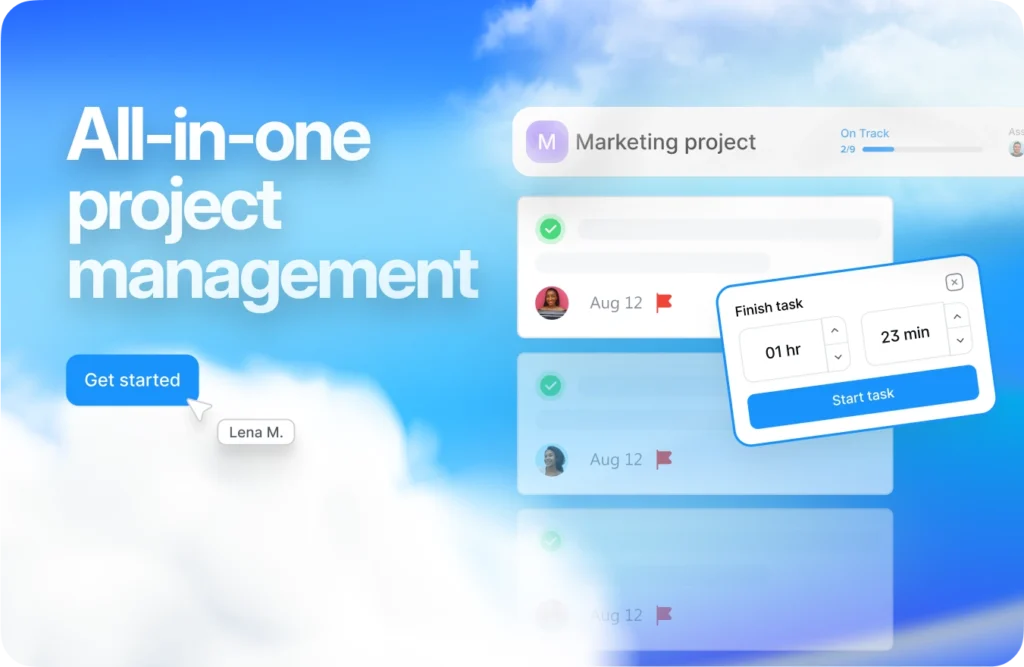
10 Major Benefits of AI in Product Management
Let’s explore the bright side—how AI is transforming product management for the better. These aren’t just theoretical benefits; they’re real, tangible advantages teams are seeing today.
1. Improved Decision-Making with Data
AI thrives on data. It doesn’t just collect it—it makes sense of it. Product managers can analyze customer behavior, market trends, and usage metrics in ways that would take humans weeks. With AI, decisions aren’t based on gut instinct alone; they’re backed by solid, real-time data.
Imagine you’re trying to decide between two product features. AI can show you which one aligns more with customer needs, market demand, and business goals—making your choice smarter and less risky.
2. Faster Product Iteration Cycles
Remember the old days when product iterations took months? With AI in the mix, iteration is becoming rapid-fire. Tools can automatically analyze feedback, identify bugs, and even simulate user behavior to predict outcomes before implementation. This accelerates development cycles and reduces time-to-market.
3. Enhanced Customer Insights
AI breaks down mountains of feedback from emails, reviews, chats, and surveys. Instead of skimming a few dozen comments, AI tools can comb through thousands in minutes, categorizing sentiment, flagging urgent issues, and spotting trends you never even considered.
This gives product managers a 360-degree view of what customers truly think, want, and need.
4. Smarter Prioritization
AI can weigh various factors—customer value, development cost, strategic fit, and urgency—to recommend which features should come next. No more endless debates or guesswork. With AI-driven frameworks, your prioritization becomes data-backed and transparent.
5. Automated Reporting
Monthly performance reports? AI’s got it covered. It can generate visual dashboards and plain-language summaries of product performance, helping stakeholders understand what’s working and what’s not—without you spending hours in spreadsheets.
6. Predictive Analytics
One of AI’s most game-changing capabilities is predicting future trends based on historical data. Predictive analytics allows product managers to forecast user behavior, churn rates, and even the success potential of new features. Imagine launching a new product update and already knowing how your users might react—that’s the power of predictive analytics.
For example, based on past interactions and engagement levels, AI can alert you if a certain user segment is likely to drop off. This insight allows your team to proactively introduce retention strategies, update onboarding flows, or even tweak features before it’s too late. You’re not just reacting to issues—you’re staying a step ahead.
Predictive analytics also help with inventory planning, resource allocation, and demand forecasting. In short, AI doesn’t just help you understand the now; it helps you plan for what’s next.
7. Improved Personalization
In today’s digital world, personalization isn’t a luxury—it’s an expectation. AI allows product teams to deliver hyper-personalized experiences by learning from individual user behaviors and preferences. This could mean personalized dashboards, content recommendations, or even customized feature sets.
Netflix, Amazon, and Spotify aren’t just popular because of their content—they’re winning because they know their users inside out. Their recommendation engines are powered by AI, constantly learning what each user loves and surfacing more of that.
Now, product managers in other industries can bring that same level of personalization to their platforms—thanks to AI.
8. Reduced Manual Workload
From automating bug triage to generating user reports and sorting feedback, AI helps offload repetitive and time-consuming tasks. This frees up product managers to focus on strategic thinking, vision alignment, and stakeholder engagement.
Think about all the hours spent manually tagging support tickets or categorizing customer feedback. AI can do this at scale and with accuracy—saving teams hundreds of hours over the course of a product cycle.
This isn’t just about convenience; it’s about letting product managers do what they do best—solve problems, build visions, and drive product success.
9. Real-Time User Feedback Analysis
Real-time feedback can be a game changer—especially when launching new features or products. AI tools can monitor user interactions, collect sentiment, and flag issues as they arise. Instead of waiting weeks to gauge user reaction, product teams get instant insight.
With tools like Qualtrics or Pendo, you can deploy in-app surveys, and AI will immediately interpret the feedback, highlighting trends and anomalies. It’s like having a team of analysts working around the clock to keep your finger on the pulse of your users.
This agility means faster responses, better user satisfaction, and fewer fires to put out post-launch.
10. Competitive Edge in Innovation
Lastly, using AI in product management gives your team a leg up. Companies leveraging AI are often more agile, more responsive, and more aligned with user needs. They can identify emerging trends faster, pivot more effectively, and launch features that resonate better with their audience.
When used correctly, AI becomes a strategic advantage—not just a technical one. It helps companies stay ahead of the curve and make smarter bets, faster.
10 Key Disadvantages of AI in Product Management
Of course, it’s not all rainbows and sunshine. Let’s take an honest look at the darker side of AI in product management. As much as it offers, there are real risks, costs, and limitations to be aware of.
1. Dependence on Data Quality
AI is only as good as the data it’s fed. If your input data is biased, outdated, incomplete, or inaccurate, the AI’s output will reflect that. It’s the classic “garbage in, garbage out” scenario.
For instance, if a product team relies on AI to analyze customer feedback, but the data is only pulled from one region or customer type, it could lead to flawed conclusions. That’s dangerous when you’re using that data to shape product strategy.
Ensuring clean, diverse, and relevant data is a big task—and many teams aren’t fully equipped to handle it.
2. Loss of Human Intuition in Decision-Making
AI is analytical, but it lacks human intuition, empathy, and creative problem-solving. Over-relying on AI could mean missing out on those gut-instinct decisions that often drive innovation and user empathy.
Think about Steve Jobs—he didn’t use user data to invent the iPhone. He imagined what users didn’t even know they wanted. That level of visionary thinking can’t be replicated by algorithms.
So while AI offers insights, it shouldn’t be the final voice in the room. Human judgment still matters—sometimes more than data.
3. High Implementation Costs
AI isn’t plug-and-play. Implementing AI into your product workflow can be expensive. Costs include:
- Purchasing or subscribing to tools
- Hiring data scientists or AI specialists
- Training the team
- Custom integrations and maintenance
For startups or smaller product teams, this can be a major hurdle. The return on investment might be significant, but the upfront costs are often intimidating.
4. Privacy Concerns
With great data comes great responsibility. AI systems often require access to sensitive user data to function effectively. This raises serious privacy and compliance concerns.
Whether it’s GDPR in Europe or CCPA in California, companies must ensure their AI tools handle data responsibly. A single misstep could lead to legal issues, loss of customer trust, or even massive fines.
Privacy-first design and ethical data usage are non-negotiable when using AI in product management.
Continuing the article…
5. Risk of Bias in Algorithms
AI systems learn from historical data—but if that data carries bias, so will the AI. This can result in flawed outputs, unfair prioritizations, or skewed product decisions. For example, if past data shows a certain demographic as less engaged, AI might deprioritize features for them, perpetuating inequality.
This becomes especially dangerous when product decisions impact diverse user groups. The lack of inclusivity or unintended discrimination can not only alienate users but also lead to reputational damage and ethical backlash.
To combat this, teams need to constantly audit AI models, diversify their datasets, and ensure that outputs align with ethical and inclusive principles.
6. Lack of Transparency (“Black Box” Issue)
Ever heard of the term “black box AI”? It refers to the fact that many AI models—especially deep learning systems—make decisions in ways that are hard to explain. This lack of transparency can be problematic for product managers who need to justify their strategies to stakeholders.
If AI recommends prioritizing Feature A over Feature B, but no one can explain why, it weakens confidence and decision-making clarity. In industries like healthcare or finance, where compliance and traceability are crucial, this opacity can be a serious liability.
Clear documentation, model explainability, and human oversight are essential to build trust in AI outputs.
7. Over-Automation
Just because you can automate something doesn’t mean you should. Over-automation can create a cold, robotic user experience and make teams too dependent on algorithms. This leads to situations where the human touch is lost—something users still crave.
Think of automated customer support chats that misinterpret problems or recommendation engines that feel repetitive. These experiences frustrate users and damage brand perception.
A balanced approach—automation where it enhances efficiency, and human interaction where empathy matters—is the smarter route.
8. Resistance from Team Members
Not everyone welcomes AI with open arms. For some team members, AI may feel like a threat—either to their jobs, expertise, or autonomy. This resistance can slow down implementation, reduce collaboration, and create friction within the product team.
It’s crucial for product leaders to manage this change effectively, providing clear communication about AI’s role as a tool, not a replacement. Training, involvement in AI processes, and transparency about how decisions are made can help reduce fear and increase adoption.
9. Ethical Concerns
With AI comes a wave of ethical considerations. Should algorithms make decisions that impact people’s lives? How do we ensure fairness? What happens if AI causes harm?
From data sourcing to decision logic, ethical AI use in product management is still a gray area. Teams need to develop internal ethics guidelines, involve diverse perspectives, and constantly evaluate the impact of AI-driven features on different user groups.
Neglecting ethics isn’t just risky—it’s irresponsible.
10. Limited Creativity in Ideation
AI can analyze what exists—but it struggles to create something truly new. Product ideation often requires lateral thinking, storytelling, and imagination—qualities that AI hasn’t mastered yet.
If product managers rely too heavily on AI for ideation, they risk becoming reactive rather than innovative. AI might suggest improvements, but groundbreaking ideas often stem from human creativity, intuition, and cultural context.
So while AI can support the process, the spark of genius still belongs to us.
AI in product management is a double-edged sword. On one side, it delivers speed, insights, automation, and precision that elevate how products are built, launched, and improved. On the other, it raises concerns about bias, costs, over-reliance, and ethical use.
The smartest product teams are those who view AI as a partner, not a replacement. It’s a tool to supercharge human creativity—not to override it.
So, should you embrace AI in your product workflows? Absolutely. But do so mindfully. Know its limits. Balance machine efficiency with human empathy. Use it to eliminate busywork—but never let it replace the soul of your product: the human touch.